Advanced SEO Techniques: Leveraging Secondary Dimensions for Strategic Benefit
Wiki Article
Discover Deeper Insights With Secondary Measurements
Second measurements serve as an effective tool in the world of data evaluation, supplying a nuanced viewpoint that goes beyond surface-level observations. Keep tuned to find just how second measurements can change the means you translate and leverage your data for critical benefits.Advantages of Additional Dimensions
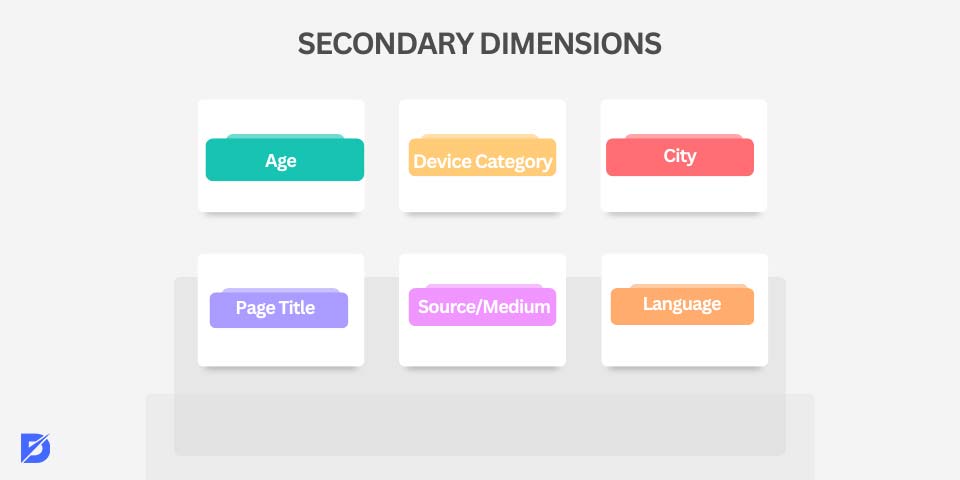
Among the essential advantages of secondary dimensions is the capability to enhance the context of the primary information. This included context makes it possible for experts to attract even more precise conclusions and make educated decisions based on a much more thorough sight of the information. Additional dimensions help in offering a much more alternative sight of the connections in between various variables, therefore helping in the recognition of underlying variables that may affect the key dataset.
Essentially, second measurements play a vital duty in improving data evaluation procedures, supplying a more nuanced viewpoint that can lead to actionable referrals and important understandings.
Execution Tips for Second Measurements
Applying second dimensions efficiently requires a calculated technique that lines up with the particular objectives of the data analysis process. To start, it is important to plainly specify the objectives of the evaluation and identify the vital metrics that will supply the most useful understandings - secondary dimensions. Select additional dimensions that complement the primary measurements and aid in revealing deeper patterns or correlations within the informationWhen carrying out additional dimensions, it is important to consider the context in which the analysis will be performed. Recognizing the audience and their information demands will certainly guide the choice of relevant additional measurements that add purposeful context to the key information points. Furthermore, make sure that the secondary dimensions chosen are compatible with the key dimensions and can be efficiently contrasted or incorporated to draw out useful insights.
Furthermore, it is advised to evaluate different mixes of second and main measurements to explore various perspectives and discover hidden relationships within the data. On a regular basis reviewing and fine-tuning the selection of additional measurements based on the evolving analytical demands will certainly make certain the evaluation remains appropriate and insightful.
Studying Data With Additional Dimensions

When evaluating data with second dimensions, it is vital to consider just how different variables interact with one an additional. By cross-referencing primary data with second dimensions, analysts can uncover relationships and dependences that use a more all natural sight of the data. This method not only improves the precision of insights but helpful site likewise aids in making even more educated decisions based on the searchings for.
Moreover, examining information with additional measurements allows the identification of outliers or abnormalities that might affect the overall evaluation. By delving deeper right into the data through secondary dimensions, analysts can get a much more profound understanding of the hidden elements driving the patterns observed in the key dataset.
Maximizing Insights With Secondary Dimensions
To draw out a higher level of depth and accuracy from information evaluation, leveraging additional measurements is critical for maximizing insights. By incorporating additional measurements right into your analysis, you can discover important partnerships and patterns that may not be instantly apparent when considering information with a primary measurement alone. Additional measurements enable you to cut and dice your information better, offering an extra extensive understanding of the elements influencing your metrics.When used properly, secondary dimensions can boost the context of your main information, offering a much more nuanced Discover More Here point of view on your analysis. As an example, by including second measurements such as time, location, or user demographics, you can get a much deeper understanding of just how different sections connect with your material or products.
Moreover, additional measurements can aid you identify outliers, fads, and correlations that might otherwise go unnoticed. By discovering your information from multiple angles, you can extract richer understandings and make even more enlightened choices based on a thorough understanding of the underlying factors at play.
## Typical Blunders to Avoid When Using Secondary Measurements
When including secondary dimensions into information analysis, it is essential to be mindful of typical errors that can impede the extraction of beneficial understandings. One widespread error is the misuse of additional dimensions without a clear purpose in mind. It is essential to define details objectives and questions before selecting secondary dimensions to guarantee they line up with the analysis purpose.
Another mistake to stay clear of is overcomplicating the analysis by consisting of way too many additional dimensions simultaneously. This can bring about information overload and make it testing to draw purposeful final thoughts from the information. It is a good idea to begin with a couple of pertinent secondary dimensions and gradually incorporate much more as required.
Moreover, neglecting data integrity concerns can dramatically impact the precision of understandings stemmed from second dimensions. Inaccurate or incomplete visit this website information can misshape the evaluation results and misguide decision-making processes. Regularly validating and cleaning up the information is essential to make sure the dependability of the understandings created.
Conclusion
Finally, the strategic utilization of secondary dimensions in data evaluation supplies a powerful device for unlocking deeper understandings and improving decision-making procedures. By integrating extra layers of details, experts can get an extra comprehensive understanding of their dataset, discover hidden patterns, and determine essential factors affecting results. With careful factor to consider and execution of secondary measurements, researchers can maximize the value of their data and drive educated decision-making in various areas.Select additional dimensions that match the main measurements and aid in discovering much deeper patterns or correlations within the data.
In addition, ensure that the secondary measurements picked are suitable with the key dimensions and can be efficiently compared or combined to extract important understandings.
Utilizing second measurements in information analysis improves the depth and breadth of insights acquired from the key information factors. By cross-referencing main information with secondary measurements, experts can uncover correlations and reliances that use a more holistic sight of the data. By including second dimensions into your evaluation, you can reveal important relationships and patterns that might not be quickly apparent when looking at data through a primary dimension alone.
Report this wiki page